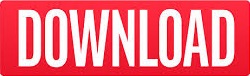
The goal is to follow a predetermined route as fast as possible avoiding collisions and road departures in a dynamic urban environment in simulation. Figure 1 shows the framework overview that has been developed in this work. More specifically, two different approaches will be developed, the Deep Q-Network (DQN) and the Deep Deterministic Policy Gradient (DDPG). In this paper, we study the inclusion of AI techniques into the control layer, referred to classic AV control architecture, through implementation of a control based on DRL algorithms for autonomous vehicle navigation.
Opendrive atmoous vehicle how to#
In terms of autonomous driving, DRL approaches have been developed to learn how to use the AV sensor suite on-board the vehicle. DRL based algorithms have been recently used to solve Markov Decision Processes (MDPs), where the scope of the algorithm is to calculate the optimal policy of an agent to choose actions in an environment with the goal of maximize a reward function, obtaining quite successful results in fields like solving computer games or simple decision-making system. In this context, AI is expanding through AV architecture, dealing with different processes such as detection, Multi-Object Tracking (MOT) and environment prediction, or evaluating the current situation of the ego-vehicle to conduct the safest decision, for example making use of DRL algorithms for behavioural driving. In that sense, a large number of classic controllers as have been successfully implemented in AV architectures.

A well defined control layer makes the vehicle robust regardless the varying environment situations, such as the traffic participants, weather conditions or traffic scenario, on the premise of guarantying vehicle stability and covering the route provided by any global planner, assuming that the control layer is based on a previous mapping and path planning layer that loads the map and planes the route. Ĭonsidering a typical AV architecture, the control layer consists of a set of processes that implements the vehicle control and navigation functionality. LIDAR-based detection methods provide accurate depth information and obtain robust results in location, object detection and scene understanding while camera-based methods provide much more detailed semantic information.
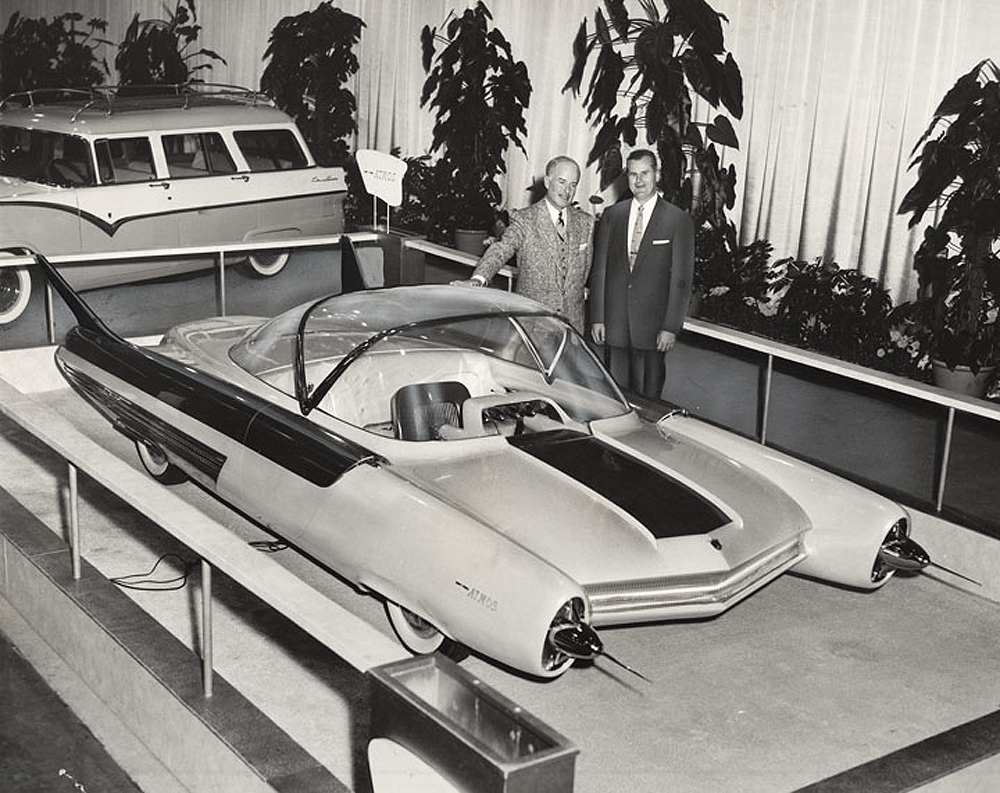
Nowadays, most self-driving vehicles are geared up with multiple high-precision sensors such as LIDAR and cameras. The teams participating in these events have demonstrated numerous technical frameworks for autonomous driving. In the last decade, various challenges, such as the well-known DARPA Urban Challenge and the Intelligent Vehicle Future Challenge (IVFC) have proven that autonomous driving can be a reality in the near future. In recent years, autonomous driving plays a pivotal role to solve traffic and transportation problems in urban areas (traffic congestions, accidents, etc) and it is going to change the way of travelling in our world in the future.
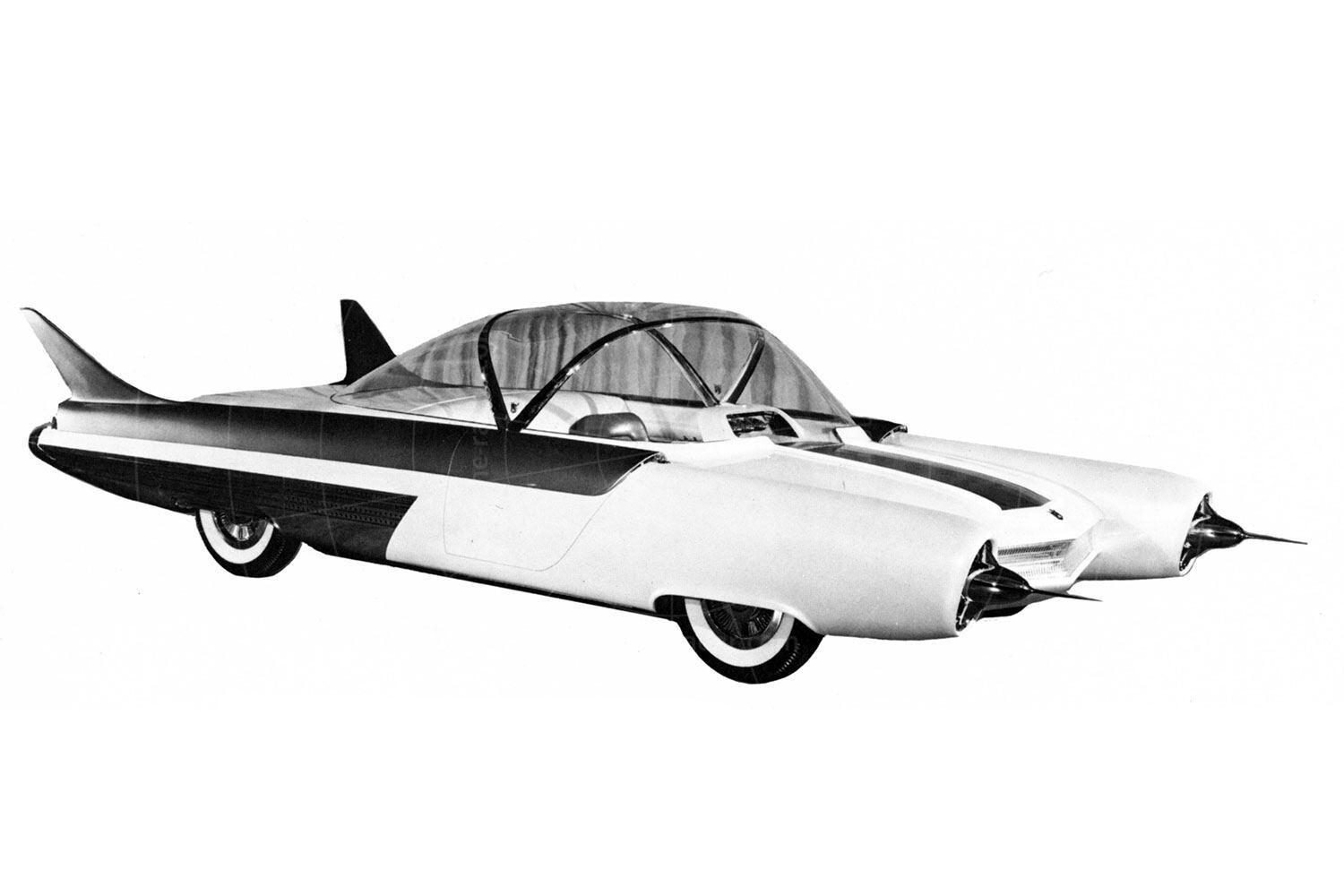
To conclude, some conclusions and future works are commented. In both cases RMSE is lower than 0.1m following trajectories with a range 180-700m. DDPG perfoms trajectories very similar to classic controller as LQR. The results obtained show that both DQN and DDPG reach the goal, but DDPG obtains a better performance.
Opendrive atmoous vehicle simulator#
For this purpose, an open-source simulator such as CARLA is used, providing to the system with the ability to perform a multitude of tests without any risk into an hyper-realistic urban simulation environment, something that is unthinkable in the real world.
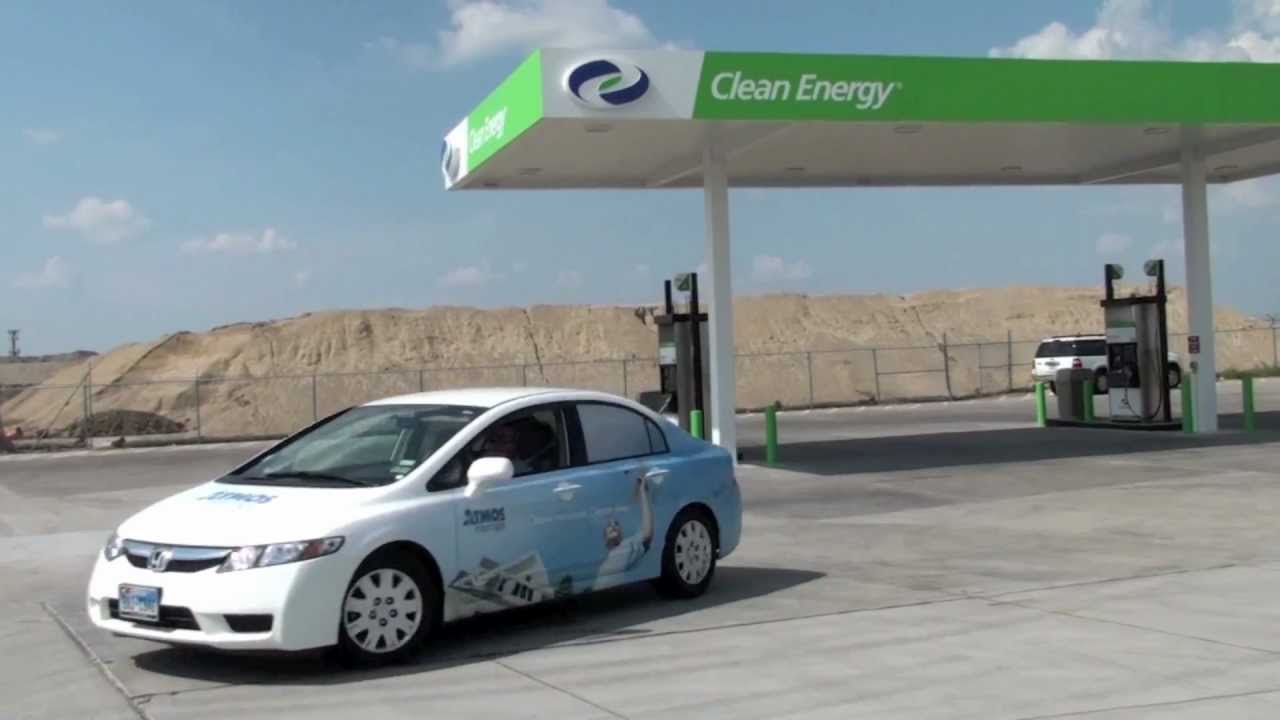
In addition, for each of the algorithms, several agents are presented as a solution, so that each of these agents uses different data sources to achieve the vehicle control commands. The aim of this work is to obtain a trained model, applying a DRL algorithm, able of sending control commands to the vehicle to navigate properly and efficiently following a determined route. More specifically, Deep Reinforcement Learning (DRL) algorithms such as Deep Q-Network (DQN) and Deep Deterministic Policy Gradient (DDPG) are implemented in order to compare results between them. This paper proposes the using of algorithms based on Deep Learning (DL) in the control layer of an autonomous vehicle. Nowadays, Artificial Intelligence (AI) is growing by leaps and bounds in almost all fields of technology, and Autonomous Vehicles (AV) research is one more of them.
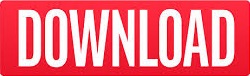